学术沙龙主题: Modeling and Learning on High-Dimensional Matrix-Variate Sequences
报告人: 张旭,华南师范大学优聘副教授
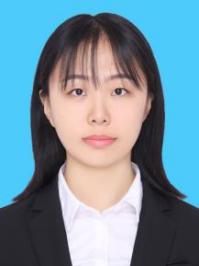
报告时间: 2025年4月2日(周三);上午10:30—12:00
报告地点:南校区网络安全大楼 120 会议室
报告人简介:张旭,华南师范大学数学科学学院优聘副教授,博士毕业于东北师范大学统计系。曾赴香港大学、香港理工大学从事博士后工作。研究方向包括网络数据分析,张量/矩阵数据的统计建模与推断。论文发表于Journal of the American Statistical Association, Statistica Sinica, Science China Mathematics等期刊。
报告摘要: We propose a new matrix factor model, named RaDFaM, which is strictly derived from the general rank decomposition and assumes a high-dimensional vector factor model structure for each basis vector. RaDFaM contributes a novel class of low-rank latent structures that trade off between signal intensity and dimension reduction from a tensor subspace perspective. Based on the intrinsic separable covariance structure of RaDFaM, for a collection of matrix-valued observations, we derive a new class of PCA variants for estimating loading matrices, and sequentially the latent factor matrices. The peak signal-to-noise ratio of RaDFaM is proved to be superior in the category of PCA-type estimators. We also establish an asymptotic theory including the consistency, convergence rates, and asymptotic distributions for components in the signal part. Numerically, we demonstrate the performance of RaDFaM in applications such as matrix reconstruction, supervised learning, and clustering, on uncorrelated and correlated data, respectively.